OpenAI's release of the GPT-4.1 family (including GPT-4.1, GPT-4.1 Mini, and GPT-4.1 Nano) marks a significant leap forward from GPT-4o, especially for developers leveraging the API. These models boast enhanced capabilities in coding, long context processing (up to 1 million tokens!), and crucially, instruction following. However, this improved adherence to instructions means that getting the best results requires adapting your prompting strategies.
Unlike previous models that might infer user intent more liberally, GPT-4.1 models follow instructions much more *literally*. This precision is powerful but demands greater clarity and explicitness in your prompts. Prompts that worked perfectly with GPT-4o might need adjustments for GPT-4.1.
This guide distills the key recommendations from OpenAI's official GPT-4.1 prompting documentation, providing actionable strategies to help you harness the full potential of these advanced AI models. We'll cover essential techniques for agentic workflows, long context handling, chain-of-thought reasoning, and precise instruction control.
Why Prompting GPT-4.1 Requires a New Approach
The core difference lies in GPT-4.1's training focus: literal instruction following. While this makes the model highly steerable and responsive to well-crafted prompts, it also means ambiguity or implicit assumptions in your instructions are less likely to be interpreted as intended. If the model's behavior isn't what you expect, often a single, clear sentence reinforcing your desired output is enough to correct its course.
Key implications for prompt engineering include:
- Be Explicit: Clearly state what the model should *do* and *not do*. Rely less on the model inferring implicit rules.
- Prompt Migration May Be Needed: Existing prompts optimized for GPT-4o or other models might underperform without modification, as GPT-4.1 will follow those instructions more strictly, potentially exposing previously overlooked flaws or assumptions.
- Increased Control: The upside is greater control over the output format, tone, reasoning process, and task execution when instructions are precise.
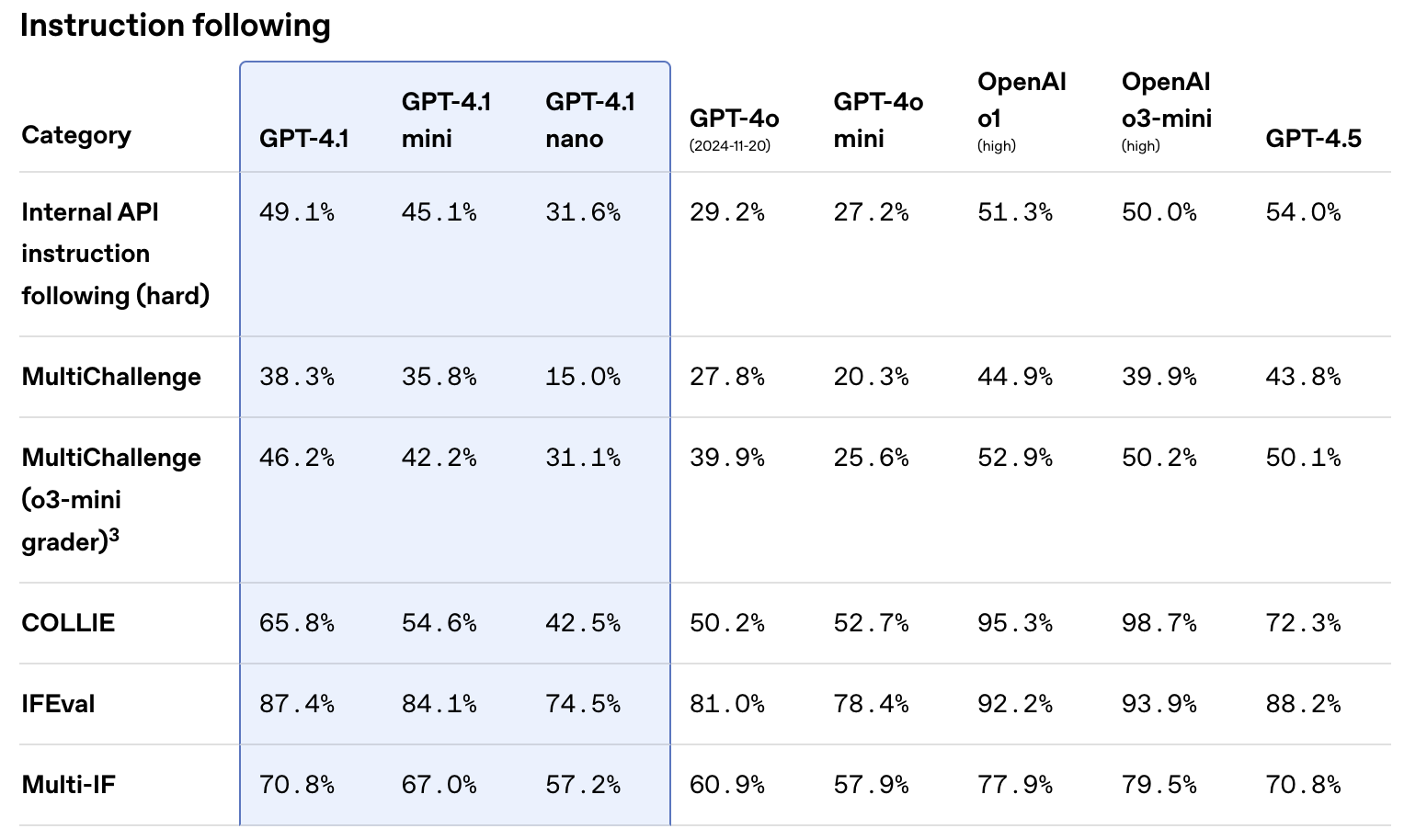
Key Prompting Strategies for GPT-4.1 Success
Based on OpenAI's extensive testing, here are the most impactful strategies for prompting the GPT-4.1 family:1. Building Robust Agentic Workflows
GPT-4.1 excels at agentic tasks where the model needs to perform multi-step operations, often involving tool use. To maximize its effectiveness as an agent:
System Prompt Essentials:
Include these reminders in your agent's system prompt:
- Persistence: Instruct the agent to continue working until the task is fully resolved before yielding control (e.g., "You are an agent - please keep going until the userâs query is completely resolved...").
- Tool-Calling Encouragement: Remind the agent to use its tools to gather information rather than guessing (e.g., "If you are not sure about file content... use your tools... do NOT guess...").
- Planning (Optional but Recommended): If you want explicit reasoning, instruct the agent to plan and reflect between actions (e.g., "You MUST plan extensively before each function call..."). OpenAI found this increased success rates on complex tasks like SWE-bench.
Effective Tool Use:
- Use the `tools` API Field: Pass tool definitions via the dedicated `tools` parameter in the API request, not by injecting schemas into the prompt text. This minimizes errors.
- Clear Naming & Descriptions: Name tools and parameters clearly. Provide detailed descriptions in the `description` field.
- Examples for Complex Tools: For intricate tools, provide usage examples within an `# Examples` section in your system prompt, rather than cluttering the tool description itself.
2. Mastering the 1 Million Token Long Context
GPT-4.1's massive context window opens up possibilities for processing extensive documents, codebases, or conversations. To optimize long context performance:
- Instruction Placement is Crucial: For best results, place your core instructions *both* at the beginning (before the context) *and* at the end (after the context). If you can only include instructions once, placing them *before* the context is generally more effective than placing them after.
- Tune Context Reliance: Explicitly tell the model whether to rely *only* on the provided context or if it can integrate its internal knowledge.
- Strict Context: "Only use the documents in the provided External Context... If you don't know the answer based on this context, you must respond 'I don't have the information needed...'"
- Allow Internal Knowledge: "By default, use the provided external context... but if other basic knowledge is needed... you can use some of your own knowledge..."
- Performance Considerations: While performance is strong up to 1M tokens (e.g., on needle-in-a-haystack tests), complex reasoning requiring synthesis across the *entire* context, or retrieving many distinct items, can still be challenging.
3. Inducing Effective Chain-of-Thought (CoT)
GPT-4.1 doesn't have built-in chain-of-thought like some "reasoning" models, but you can easily prompt it to "think out loud," breaking down problems step-by-step. This improves quality on complex tasks, albeit with slightly higher latency and cost.
- Basic CoT Prompt: Start by adding a simple instruction at the end of your prompt, like: "First, think carefully step by step about... Then, ..."
- Refine Based on Failures: Analyze where the model's reasoning goes wrong in your specific use case. Add more explicit instructions to guide its planning and analysis process.
- Structured Reasoning Example: For more complex scenarios, consider providing a defined reasoning strategy:
# Reasoning Strategy 1. Query Analysis: Break down and analyze the query... 2. Context Analysis: Carefully select and analyze relevant documents... a. Analysis: ... b. Relevance rating: [high, medium, low, none] 3. Synthesis: summarize which documents are most relevant... # User Question {user_question} # External Context {external_context} First, think carefully step by step... adhering to the provided Reasoning Strategy. Then...
4. Leveraging Precise Instruction Following
GPT-4.1's literalness is a superpower for controlling output format, tone, style, and adherence to specific rules.
Recommended Workflow:
- Start with a general `# Instructions` or `# Response Rules` section.
- Add specific sub-sections (e.g., `# Output Format`, `# Sample Phrases`) for detailed control.
- If a specific workflow is needed, provide an ordered list of steps.
- Debug by checking for conflicting or underspecified instructions. Remember, instructions closer to the end of the prompt often take precedence.
- Use clear examples (`# Examples`) demonstrating the desired behavior, ensuring rules mentioned are reflected in examples.
- Avoid relying heavily on ALL CAPS or "tips/bribes" initially; use them sparingly if needed, as GPT-4.1 might follow them *too* strictly.
Common Pitfalls & Mitigations:
- Overly Strict Rules: Telling a model it *must always* do something (e.g., call a tool) can lead to hallucinated inputs if information is missing. Add caveats: "...if you donât have enough information... ask the user..."
- Repetitive Phrasing: Models might overuse provided sample phrases verbatim. Instruct it to "vary the sample phrases as necessary".
- Excessive Verbosity: Without guidance, models might add extra prose. Instruct conciseness or provide formatting examples.
General Prompting Best Practices for GPT-4.1
Beyond the specific strategies above, keep these general tips in mind:Recommended Prompt Structure:
Start with a structure like this, adapting as needed:
# Role and Objective
# Instructions
## Sub-categories for more detailed instructions (e.g., Output Format, Tone)
# Reasoning Steps (Optional: Guide CoT)
# Output Format (If specific structure is needed)
# Examples
## Example 1
# Context (Provide relevant documents, history, etc.)
# Final instructions and prompt to think step by step (Place user query/task here)
Choosing Effective Delimiters:
- Markdown: Recommended starting point. Use headings (`#`, `##`), lists, and backticks (`` `code` ``) for clarity.
- XML Tags: Perform well, especially for nesting (like examples within `
`) or adding metadata (` `). Useful if your content doesn't contain much XML itself. - JSON: Highly structured but can be verbose and require escaping.
- Long Context Delimiters: OpenAI found XML (`
... `) and simple structured formats (`ID: 1 | TITLE: ... | CONTENT: ...`) worked well for large document sets. JSON performed poorly in their tests for this specific use case.
Choose delimiters that provide clear structure and contrast with your main content.
Important Caveats:
- Long Repetitive Outputs: In rare cases, the model might resist generating extremely long, highly repetitive outputs (e.g., analyzing hundreds of items individually). If needed, instruct it strongly or consider breaking down the task.
- Parallel Tool Calls: While generally reliable, rare issues with parallel tool calls have been observed. Test thoroughly and consider setting `parallel_tool_calls=false` if you encounter problems.
A Quick Look at GPT-4.1 Models and Pricing
While this guide focuses on prompting, understanding the available models helps choose the right tool for the job. The GPT-4.1 family includes:
- GPT-4.1 Nano: Most cost-effective, suitable for simpler tasks like classification.
- GPT-4.1 Mini: Often cited as the sweet spot, balancing strong performance (sometimes matching the full model) with significantly lower cost and higher speed than GPT-4o.
- GPT-4.1: The flagship model, best for the most demanding tasks, multimodal capabilities, and complex reasoning over long contexts.
Here's the pricing structure (as of OpenAI's launch information, per 1 Million tokens):
Model (Prices are per 1M tokens) |
Input | Cached Input | Output |
---|---|---|---|
gpt-4.1 | $2.00 | $0.50 | $8.00 |
gpt-4.1-mini | $0.40 | $0.10 | $1.60 |
gpt-4.1-nano | $0.10 | $0.025 | $0.40 |
Conclusion: Embrace Explicit Prompting for GPT-4.1
The GPT-4.1 models represent a powerful evolution in AI capabilities, particularly for developers building sophisticated applications. Their enhanced ability to follow instructions literally offers unprecedented control but requires a shift towards more explicit, detailed, and well-structured prompts.
By applying the strategies outlined in this guideâfocusing on clear system prompts for agents, strategic instruction placement for long context, inducing chain-of-thought when needed, and leveraging precise instruction followingâyou can unlock the true potential of GPT-4.1. Remember that AI engineering is empirical; use these guidelines as a starting point, build robust evaluations, and iterate on your prompts to achieve optimal results for your specific use case.